Time-of-use pricing program for residential electricity demand in Spain reduced consumption during mid and peak hours on average, while maintaining consumption during lower-priced hours.
The growing share of renewable electricity generation, coupled with demand fluctuations, emphasizes the inherent challenge of balancing energy supply and demand. Thus, the crucial need for pricing structures that precisely capture these dynamics to prevent market inefficiencies. Several governments are recognizing the need to ensure a more effective alignment between consumer prices and the varying costs of electricity generation, transitioning from static to dynamic pricing models. The success of dynamic pricing will depend on the potential market failures and the proposed mechanism. For instance, day-ahead real-time pricing (RTP) may face challenges in the presence of imperfect information or adjustment costs, necessitating the use of automated load-shifting technologies for an effective demand response. Alternatively, predetermined retail pricing policies, exemplified by time-of-use (TOU) pricing, can address information constraints and reduce short-term costs associated with shifting consumption.
How would consumer demand response change under a policy that significantly reduces prices during off-peak hours and substantially increases prices during peak hours? In June 2021, the Spanish government implemented a regulatory change affecting system and network charges, constituting 50% of the total electricity bill. The new regulation introduced three distinct marginal prices based on the time of day and the day of the week. Workdays were categorized into peak, off-peak, and mid-peak hours, while weekends and holidays were designated as off-peak hours.
The authors estimate a Differences-in-Differences (DID) fixed effects model, comparing hourly differences in electricity consumption before and after the policy in Spain’s treated distribution areas and Portugal, the control group, including a rich set of fixed effects. They find that the program could have reduced consumption by up to 9% during peak periods. The authors then explore the potential habit formation spillover from weekdays to weekends when prices are unaffected by the policy. Differentiating between these periods, results reveal a significant demand response for mid- and peak hours, even on weekends when prices do not vary by time of day (Figure 1).
Figure 1: Policy effects by hour of the day and type of day
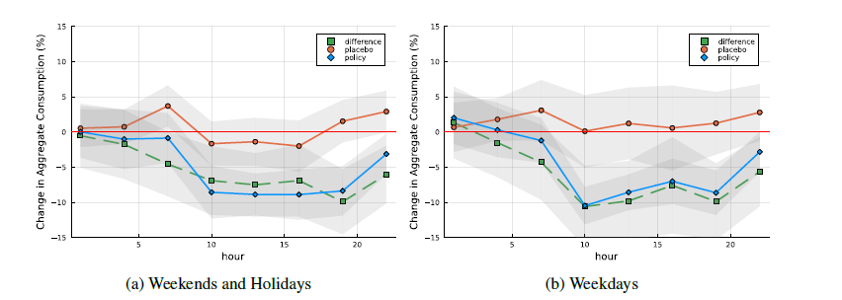
The authors then turn to Machine Learning methods to choose the correct specification in a context with many possible covariates and interactions. First, they use pre-treatment data to create a distribution area-specific electricity consumption model, that then use to create out-of-sample predictions for the post-treatment period. In a second step, the authors compute prediction errors and regress them as the dependent variable of the DID model estimated before. They find evidence that using machine learning techniques helps to reduce the sensitivity of the estimates across different fixed-effect specifications. Overall, these results align with the literature studying behavioral factors in explaining consumer responsiveness, suggesting that a predetermined pricing program, by enhancing consumer awareness, makes these prices more salient thus triggering stronger household responses.